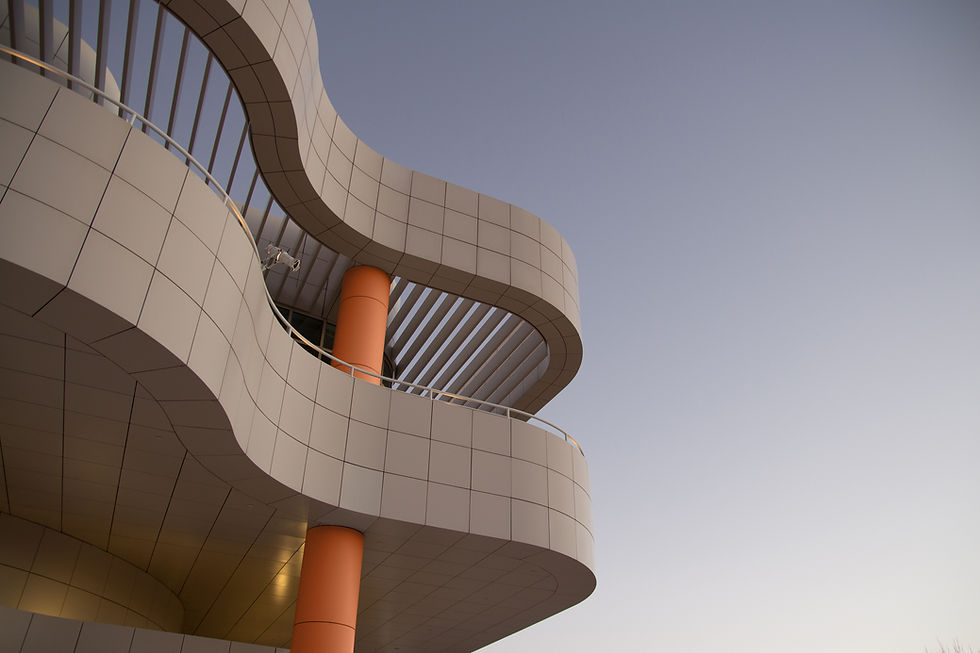
Revolutionizing Earth and Space: How AI is Transforming Environmental Monitoring and Space Weather Forecasting
Dec 5
3 min read
0
0
0
Artificial intelligence transforms science and technology, driving advancements in Earth science applications and space weather forecasting. Recent developments from NASA, IBM, Forschungszentrum Jülich, Los Alamos National Laboratory, and the University of North Carolina at Chapel Hill demonstrate how AI-powered models revolutionize data analysis, environmental monitoring, and satellite protection.
NASA, IBM, and Forschungszentrum Jülich have expanded the Prithvi Geospatial artificial intelligence foundation model, integrating global data to enhance Earth science applications. Initially released in August 2023, the model is pre-trained on NASA's Harmonized Landsat and Sentinel-2 dataset and now includes worldwide data to support applications such as tracking land use changes, monitoring natural disasters, and predicting crop yields. The enhanced model on Hugging Face enables businesses and researchers to transform NASA's scientific expertise and vast datasets into actionable insights, fostering economic and societal benefits.

Kevin Murphy, NASA's Chief Science Data Officer, emphasized that integrating global data into the Prithvi model significantly enhances its potential, enabling faster, more informed decisions. Built with self-supervised learning techniques, the model relies on a high-quality dataset representing diverse landscapes and ecosystems, ensuring reliability for environmental analysis. By addressing challenges like cloud cover in satellite imagery, the model reconstructs gaps through the Multi-Temporal Cloud Gap Imputation application, offering clear views of Earth's surface over time. Other applications include Multi-Temporal Crop Segmentation for mapping crop patterns, flood mapping to classify surface water in diverse ecosystems, and wildfire scar mapping to detail post-fire landscapes.
In addition to its current capabilities, the model has been rigorously tested with applications such as landslide detection, burn intensity estimation, and biomass estimation. Rahul Ramachandran, the AI foundation model for science lead at NASA, highlighted that user feedback had driven the updates, ensuring improved versatility and performance for various environmental monitoring tasks. This collaborative effort involved NASA's Interagency Implementation and Advanced Concepts Team, IBM Research, and the Jülich Supercomputing Centre, with the model developed on the JUWELS supercomputer.

Simultaneously, researchers at Los Alamos National Laboratory and the University of North Carolina at Chapel Hill are leveraging machine learning to improve space weather forecasting. They focus on "killer electrons," high-energy particles trapped in Earth's Van Allen belts, which pose significant risks to satellites by causing electronic malfunctions. The newly developed Predictive MeV Electron—Medium Earth Orbit (PreMevE-MEO) model combines data from 12 medium-Earth-orbit GPS satellites and one Los Alamos geosynchronous-Earth-orbit satellite. Using a sophisticated machine-learning algorithm that integrates convolutional neural networks and transformers, the model delivers accurate hourly forecasts of electron dynamics.
Yue Chen, a Los Alamos physicist and lead research author, underscored the importance of long-term space observations in the AI era. The PreMevE-MEO model utilizes Los Alamos's unique GPS data, which includes over 100 satellite years of archived X-ray dosimeter particle data categorized as big data. This model represents a critical step forward in space weather forecasting, with the potential to serve as an operational warning tool for satellites. It aligns with the U.S. National Space Weather Strategy and Action Plan, which calls for leveraging historical data from satellites and observatories to improve forecasting models for space weather events.

The applications of these AI models are vast, demonstrating their potential to address global challenges. The Prithvi model's ability to monitor environmental changes, support disaster management, and enhance agricultural planning showcases its capacity to drive societal benefits. Similarly, the PreMevE-MEO model provides a new layer of protection for satellites, ensuring more resilient space infrastructure. Both advancements highlight the growing role of artificial intelligence in interpreting massive datasets and translating them into actionable insights, driving innovation, and solving critical problems across Earth and space sciences.