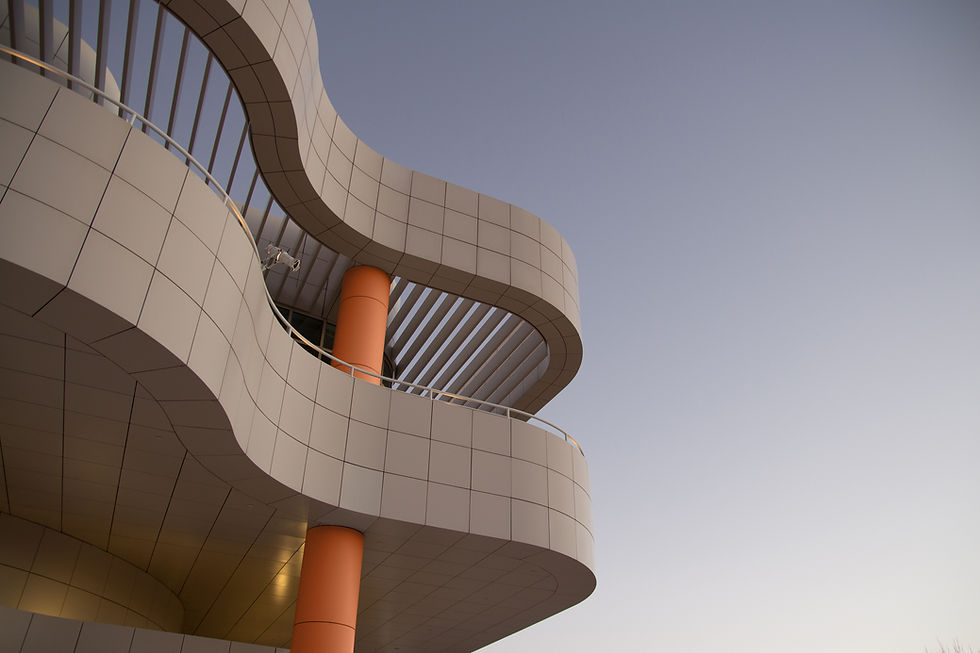
OpenAI and others seek new path to smarter AI as current methods hit limitations
Nov 21
2 min read
0
0
0
Artificial intelligence companies, including OpenAI, are navigating unexpected delays and challenges in developing ever-larger language models by pursuing training techniques that enable algorithms to adopt more human-like ways of "thinking." A group of AI scientists, researchers, and investors believe these methods, exemplified in OpenAI's recently launched o1 model, could redefine the AI arms race, impacting resource needs ranging from energy consumption to chip technology. This strategic shift reflects a growing recognition of the limits of "scaling up" through simply increasing data and computational power—a strategy that has historically driven advancements such as ChatGPT.

OpenAI and others seek new path to smarter AI as current methods hit limitations | Reuters
Ilya Sutskever, a co-founder of OpenAI who recently founded Safe Superintelligence (SSI), acknowledged a plateau in gains from scaling up pre-training, the process through which vast quantities of unlabeled data help AI models understand language structures. Sutskever, who once championed massive generative AI leaps driven by expansive datasets and computational power, now believes, "the 2010s were the age of scaling; now we're back in the age of wonder and discovery." While he remains guarded about SSI's approach to this challenge, Sutskever hinted at exploring alternatives to conventional scaling.

The push to surpass OpenAI’s GPT-4 has proven fraught for AI researchers across the industry. Developing such large models requires "training runs" that can cost tens of millions, involving complex chip configurations and consuming vast data reserves. Hardware failures and power shortages further complicate these efforts, delaying breakthroughs. As easily accessible data becomes scarce, AI experts are shifting focus to techniques like "test-time compute," where models enhance themselves during inference by generating and evaluating multiple real-time solutions. This approach, demonstrated by OpenAI’s o1 model, enables more human-like, multi-step problem-solving, even for tasks demanding sophisticated reasoning.
Noam Brown of OpenAI described how giving an AI "20 seconds to think" during a poker game achieved similar performance improvements to training it extensively over a prolonged period. Techniques like these, layered on top of foundational models such as GPT-4, represent OpenAI's strategy to maintain an edge as competitors, including Anthropic, xAI, and Google DeepMind, pursue their variations of this method.

As AI companies explore inference optimization, the market landscape is poised for disruption. Nvidia's dominance in AI chip supply has been central to its recent ascension as the world's most valuable company, surpassing Apple. However, as distributed, cloud-based inference systems gain traction, Nvidia may face increased competition. Prominent venture capitalists, including Sequoia Capital and Andreessen Horowitz, are carefully weighing the implications for their significant investments. Nvidia's CEO, Jensen Huang, highlighted demand for the company’s latest chips designed for inference processing, framing it as a "second scaling law" in AI's evolution.