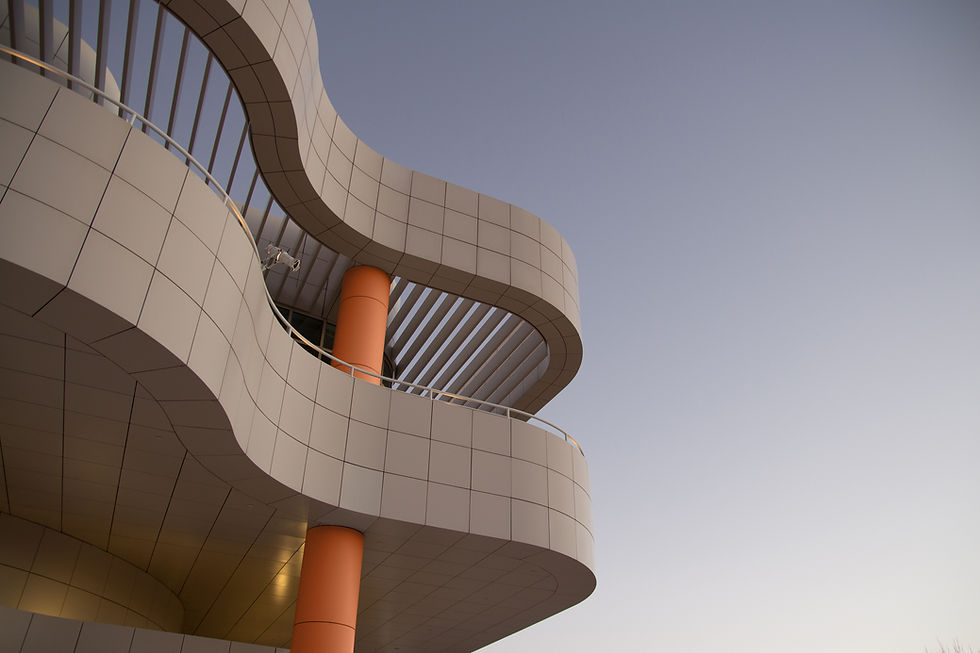
MIT’s Breakthrough Algorithm Revolutionizes AI Training for Complex Tasks
Jan 16
2 min read
0
0
0
MIT researchers have introduced a groundbreaking algorithm that significantly improves the training of AI systems for complex and variable tasks. Their innovative approach, known as Model-Based Transfer Learning (MBTL), not only enhances AI reliability but also achieves up to a 50-fold increase in efficiency, marking a major advancement in the field of reinforcement learning (RL). This algorithm paves the way for smarter, more adaptable, and scalable AI systems by addressing the challenges associated with traditional AI training methods.
Reinforcement learning has made strides in robotics, traffic management, and medicine. However, a persistent challenge remains: many RL models struggle to adapt when faced with slight variations in the tasks they were trained to perform. For example, an AI system designed to optimize traffic flow might falter when tasked with managing intersections with different lane configurations, speed limits, or traffic patterns. This brittleness limits the generalizability and scalability of AI systems, posing significant hurdles for real-world applications.
To tackle this issue, MIT researchers developed MBTL, an efficient algorithm that strategically selects which tasks to train on. Traditional training approaches fall into two extremes: training a separate model for each task, which yields high accuracy but demands enormous computational resources, or training a single model for all functions, which is more efficient but often leads to subpar performance. MBTL strikes a balance by focusing on a subset of tasks most likely to improve overall performance, reducing training costs while maintaining effectiveness.

The algorithm models two critical factors: how well an AI performs when trained on a single task and how much that performance degrades when applied to a different task—a concept known as generalization performance. By explicitly modeling these factors, MBTL identifies the most promising tasks to train on, prioritizing those that maximize performance improvements. The algorithm sequentially adds tasks, ensuring the best marginal gains in efficiency and reliability.
When tested on simulated environments, including traffic signal control and speed advisory systems, MBTL proved five to 50 times more efficient than traditional methods. For instance, while standard approaches required data from 100 tasks, MBTL achieved the same performance by training on just two tasks. This dramatic efficiency reduces computational costs and accelerates the training process. According to Cathy Wu, a senior researcher on the project, the simplicity of MBTL makes it easier for the broader AI community to adopt and implement, further amplifying its impact.
The implications of this research extend far beyond traffic management. MBTL can potentially revolutionize various domains, including autonomous robotics, medical decision-making systems, and climate modeling. The researchers aim to expand the algorithm’s capabilities to handle more intricate, high-dimensional problems, making it a cornerstone for training AI systems in increasingly complex environments.

This innovative approach offers a practical solution to AI’s most pressing challenges: ensuring robustness and adaptability in systems that impact daily life. By focusing on tasks that matter most, MBTL enhances the performance of AI systems and makes them more feasible for real-world applications. As AI continues integrating into essential systems, advancements like MBTL will be crucial for unlocking its full potential.
The MIT team is charting a new path for AI through their work, making it more innovative, reliable, and scalable. With continued development, MBTL could redefine how AI tackles complexity, driving innovation across industries and reshaping the possibilities of artificial intelligence.