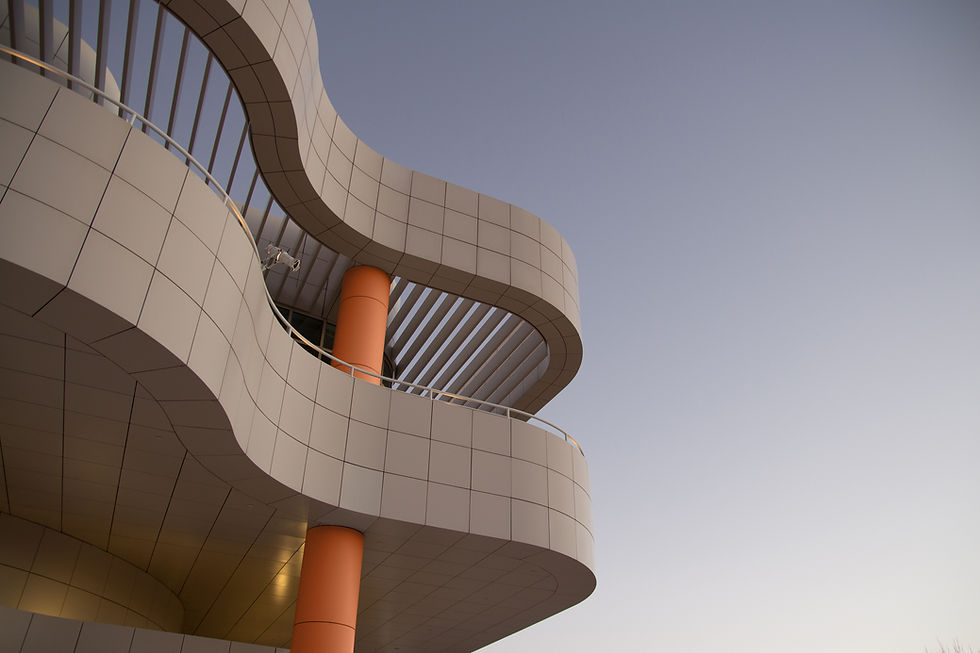
Breaking Barriers with AI: Real-Time American Sign Language Recognition
6 days ago
3 min read
0
2
0
Sign language serves as a sophisticated and essential mode of communication for individuals who are deaf or hard of hearing, relying on intricate hand movements, facial expressions, and body language to convey meaning. American Sign Language (ASL), one of many unique sign languages worldwide, exemplifies this complexity with its distinct grammar, syntax, and vocabulary. Yet communication barriers persist, mainly when ASL interpreters or translation tools are unavailable. To address this challenge, researchers have developed innovative AI-based systems that interpret ASL gestures in real-time, breaking down communication barriers and fostering inclusivity.
Researchers from the College of Engineering and Computer Science at Florida Atlantic University (FAU) recently conducted a groundbreaking study to detect and classify American Sign Language alphabet gestures using a unique combination of advanced computer vision tools and deep learning models. This pioneering system achieved remarkable precision and reliability, marking a significant milestone in assistive technology.

The research's heart was a custom dataset consisting of 29,820 static images of ASL hand gestures. These images were meticulously annotated using MediaPipe, a tool that identifies 21 key landmarks on the hand, providing detailed spatial data about its structure and positioning. This annotation process was essential to enhancing the precision of YOLOv8, a state-of-the-art deep learning model employed for object detection and classification.
By integrating MediaPipe's hand-tracking capabilities with YOLOv8's advanced object detection, the researchers significantly improved the system's ability to detect subtle variations in hand poses. This innovative two-step approach enabled the model to achieve an impressive 98% accuracy, with a recall rate of 98% and an F1 score of 99%. The model also delivered a mean Average Precision (mAP) of 98% and a mAP50-95 score of 93%, demonstrating its exceptional reliability in recognizing ASL gestures. Such metrics underline the robustness and precision of the system, particularly in real-world settings where variability in hand movements and positions can pose significant challenges.
The researchers highlighted that combining MediaPipe's spatial hand pose tracking with YOLOv8's object detection and meticulous hyperparameter fine-tuning represents a new and innovative direction for gesture recognition. Unlike previous research efforts, this approach achieves unprecedented accuracy by capturing the subtle intricacies of ASL hand gestures. Bader Alsharif, a Ph.D. candidate and first author of the study, emphasized that the model's success stems from the careful integration of landmark tracking, deep learning, and advanced dataset creation.

The study's results, published in Franklin Open, demonstrate the system's ability to detect and classify ASL gestures with minimal errors, showcasing its real-world potential. According to Mohammad Ilyas, Ph.D., co-author, and a professor at FAU, the model's ability to maintain high recognition rates even under diverse hand positions and gestures highlights its adaptability and strength. This success opens the door to practical, real-time applications where ASL recognition can significantly enhance communication accessibility for individuals who are deaf or hard of hearing.
Future efforts will focus on further expanding the dataset to include a broader range of hand shapes and gestures, improving the system's ability to differentiate between visually similar gestures. Optimizing the model for deployment on edge devices will be a priority, ensuring it can operate efficiently in resource-constrained environments without sacrificing real-time performance.
Stella Batalama, Ph.D., dean of FAU's College of Engineering and Computer Science, noted the research's transformative potential in creating tools that enhance accessibility across various settings. The model's ability to interpret ASL gestures with such precision opens up new possibilities in education, healthcare, and social interactions, offering more inclusive solutions for individuals who rely on sign language.

This study represents a significant milestone in gesture recognition by combining cutting-edge computer vision with deep learning. It contributes to the broader goal of fostering a more inclusive society where communication barriers are significantly reduced. The system's remarkable accuracy and adaptability underscore its promise as a reliable solution for real-time ASL interpretation, paving the way for meaningful advancements in assistive technology.